by Roman Taraban, Ph.D. Texas Tech University
When you read, do you ask yourself whether the material is contributing to your knowledge of the subject, whether you should revise your prior knowledge, or how you might use the new knowledge that you are acquiring? Do you highlight information or make notes in the margins to better remember and find information later on? Prior research by Pressley and colleagues (e.g., Pressley & Afflerbach, 1995) suggested that the type of metacognitions suggested by reading strategies like these were critical for effective reading comprehension.
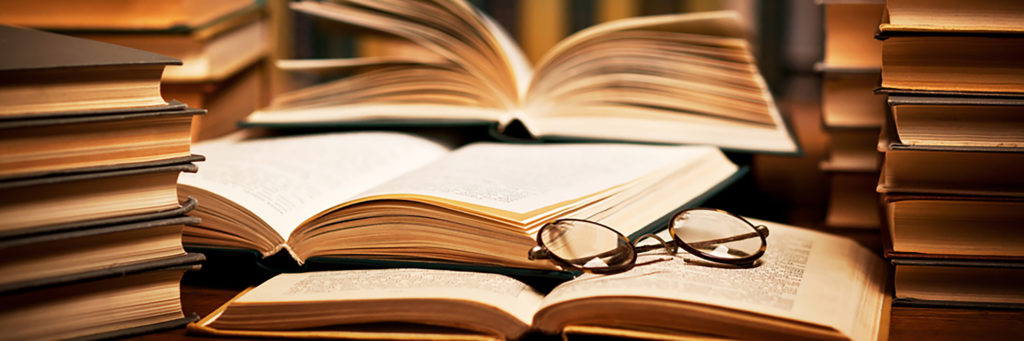
Inspired by that research, Taraban et al. (2000) conducted a study involving 340 undergraduates and 35 reading strategies like those suggested by Pressley and colleagues and found that self-reports of strategy use were significantly associated with grade-point averages (GPA). Specifically, students who reported higher use of reading strategies also had higher GPAs. Additionally, responses to open-ended questions showed that students who could name more reading strategies and reading goals also had significantly higher GPAs.
The data in Taraban et al. (2000) overwhelmingly suggested a strong positive relationship between students’ knowledge and use of reading goals and strategies and their academic performance. More generally, data by Taraban et al. and others suggest that effective reading depends on metacognitive processing – i.e., on directed cognitive effort to guide and regulate comprehension. Skilled readers know multiple strategies and when to apply them. In the remainder of this post, I review subsequent developments associated with metacognitive reading strategies, including cross-cultural comparisons, as well as raising a question about the relevance of these strategies to present-day text processing and comprehension given widespread technological developments.
Analytic VS Pragmatic Reading Strategies
In 2004, my students and I created a questionnaire, dubbed the Metacognitive Reading Strategies Questionnaire (MRSQ) (Taraban et al., 2004). The questionnaire drew on the strategies tested earlier in Taraban et al. (2000) and organized the strategies into two subscales through factor analytic methods: analytic strategies and pragmatic strategies. The analytic scale relates to cognitive strategies like making inferences and evaluating the text (e.g., After I read the text, I consider other possible interpretations to determine whether I understood the text.). The pragmatic scale relates to practical methods for finding and remembering information from the text (e.g., I try to underline when reading in order to remember the information.). Students respond to these statements using a five-point Likert-type scale: Never Use, Rarely Use, Sometimes Use, Often Use, Always Use.
Initial applications of the MRSQ suggested that the two-factor model could aid in better understanding students’ use of metacognitive comprehension strategies. Specifically, in students’ self-reports of expected GPA for the coming academic year, there was a significant positive correlation with analytic strategies but a non-significant correlation with pragmatic strategies, which suggested that students who reported higher use of analytic strategies also anticipated doing well academically in the coming academic year.
Cross-Cultural Explorations of Reading Strategies
Vianty (2007) used the MRSQ to explore difference in students’ use of metacognitive reading strategies in their native language, Bahasa Indonesia, and their second language, English. Participants were students in a teacher education program who completed the MRSQ in English and Bahasa Indonesia. Vianty found that students processed language differently in their native language compared to a non-native language.
In comparing mean use of analytic strategies when reading in their native language compared to English, Vianty found that nearly all means were higher for Bahasa Indonesia. T-tests showed significant differences favoring Bahasa Indonesia for eight out of sixteen analytic strategies. Conversely, four of the six pragmatic strategies were favored when reading English, however, only one difference (I take notes when reading in order to remember the information) was significant on a t-test. Vianty concluded that students used analytic strategies significantly more in Bahasa Indonesia than English. Conversely, use of pragmatic strategies was higher when reading in English, but the effect was weak.
Taraban et al. (2013) compared US and Indian engineering undergraduates on their application of analytic and pragmatic strategies. The language of instruction in Indian universities is English; however, this is not typically the native language (the mother tongue) of the students. Therefore, the researchers predicted lower use of analytic strategies and higher use of pragmatic strategies among Indian students compared to US students, reasoning from the findings in Vianty (2007). The latter but not former prediction was supported. Indeed, Indian students applied analytic strategies significantly more frequently than US students. Pragmatic strategy use was significantly lower than analytic strategy use for US students but not for Indian students, who applied analytic and pragmatic strategies equally often. Contrary to the findings in Vianty (2007), these findings suggest that students can make significant use of analytic and pragmatic strategies in a non-native language.
The most comprehensive cross-linguistic comparison was conducted recently by Gavora et al. (2019), who compared analytic and pragmatic strategy use, measured by variants of the MRSQ, among 2692 students from Poland, Hungary, Slovakia, and the Czech Republic, enrolled in education programs, primarily teacher and counseling. Students in Hungary, Slovakia, and the Czech Republic reported significantly higher use of pragmatic over analytic strategies. Students in Poland showed a converse preference, reporting significantly more frequent use of analytic strategies. Quite striking in the results were the significant correlations between pragmatic strategy use and GPA, and analytic strategy use and GPA, for all four countries. Specifically, the correlation showed that higher frequency use of both pragmatic and analytic strategies was associated with more successful academic performance.
Gavora et al. (2019) suggest that “In order to succeed academically, students direct their reading processes not towards comprehension but to remembering information, which is the core component of the pragmatic strategy” (p. 12). Their recommendation, that “educators’ attention should be focused on developing especially analytic strategies in students,” is strongly reminiscent of the ardor with which Pressley and colleagues began promoting metacognitive reading strategies beginning in the elementary grades.
However, given the significant correlations between both analytic and pragmatic strategy use with GPA, it may be that the predominance of analytic strategies is not what is important, but whether application of either type of strategy – analytic or pragmatic – aids students in their academic achievement. The data from Vianty (2007) may be informative in this regard, specifically, the finding that those students applied pragmatic strategies more frequently than analytic strategies when the context – reading outside their native language – dictated a more pragmatic approach to reading and comprehension.
A relevant point made by Gavora et al. relates to the samples that have been tested to-date, and the relevance of context to strategy use. They point out that in contexts like engineering (e.g., Taraban et al., (2013), the context may support more analytic thinking and analytic strategy use. The Gavora et al., sample consisted of humanities students, which, on their argument, may have resulted in an overwhelming affirmation of pragmatic strategies. Further comparisons across students in different programs is certainly warranted.
Changing Times: The Possible Influence of Technology on Reading
An additional question comes to mind, which is the effect of widespread technology in instructional settings. When I, like others, am uncertain about a definition, algorithm, theory, etc., I find it very easy to simply Google the point or look for a YouTube, which I simply need to read or watch for an explanation. This personal observation suggests that perhaps the strategies that are probed in the MRSQ may, at this point, be incomplete, and in some instances, somewhat irrelevant. The next step should be to ask current students what strategies they use to aid comprehension. Their responses may lead to new insights into contemporary student metacognitions that assist them in learning.
In conclusion, there is no doubt that metacognitive strategies are essential to effective information processing. However, there may be room to reconsider and update the strategies that students employ when reasoning and searching for information and insights to guide and expand comprehension and learning. It may be that current technology has made students more pragmatic and a promising goal for further research would be to uncover the ways in which that pragmatism is being expressed through new search strategies.
References
Gavora, P., Vaculíková, J., Kalenda, J., Kálmán, O., Gombos, P., Świgost, M., & Bontová, A. (2019). Comparing metacognitive reading strategies among university students from Poland, Hungary, Slovakia and the Czech Republic. Journal of Further and Higher Education, 1-15.
Pressley, M., & Afflerbach, P. (1995). Verbal protocols of reading: The nature of constructively responsive reading. Hillsdale, NJ: Erlbaum.
Taraban R, Kerr M, Rynearson K (2004) Analytic and pragmatic factors in college students’ metacognitive reading strategies. Reading Psychology, 25(2), 67–81.
Taraban, R., Rynearson, K., & Kerr, M. (2000). College students’ academic performance and self-reports of comprehension strategy use. Reading Psychology, 21, 283–308.
Taraban, R., Suar, D., & Oliver, K. (2013). Information literacy of US and Indian engineering undergraduates. SpringerPlus, 2(1), 244.
Vianty, M. (2007). The comparison of students’ use of metacognitive reading strategies between reading in Bahasa Indonesia and in English. International Education Journal,8(2), 449–460.