by Roman Taraban, Ph.D., Texas Tech University
Cognition involves many kinds of processes. There is categorization, problem solving, decision making, and comprehension, among others. Metacognition may involve these processes, but is different from them. Metacognitive thinking is thinking about the processes themselves (Draeger, 2015). That is, thinking about the processes involved in categorization, comprehension, and so on, and how these processes relate to one’s information processing capabilities. John Flavell, who coined the term metacognition, suggested that metacognitive processing relates not only to the individual thinkers but to others as well: “Metacognitive knowledge is one’s stored knowledge or beliefs about oneself and others as cognitive agents, about tasks, about actions or strategies, and about how all these interact to affect the outcomes of any sort of intellectual enterprise” (Flavell, 1999, p. 906). Consideration of how thinking in another person informs one’s own metacognitive knowledge is seldom considered in discussions of metacognition. In this post, I relate how reflecting on how others process information, specifically, how machines process information, can inform a person’s understanding of how he or she processes information. The metacognitive processes of interest here are those related to language processing, and the specific machine processing relates to that of machine systems called chatbots.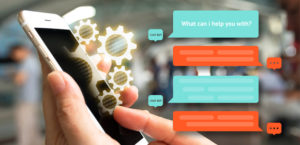
Chatbots are computer programs that interact with a person auditorily or through text. They are designed to communicate as much as possible like humans, in order to convey a sense of natural language communication. Chatbots are typically developed for commercial purposes, to provide customer service, for instance, or information about products or places. You will find chatbots on websites for companies, organizations, and events.
Recently I taught a graduate seminar on psycholinguistics, which is concerned with language acquisition, production, and comprehension. I assigned students the task of building chatbots for an application that interested them, for instance, a chatbot that could inform a user of the movies currently playing around town and show times. After students had built their chatbots and demonstrated them to the class, I assigned a written take-home metacognitive activity in which students had to discuss some aspect of the nature of chatbot language, for example, ways in which chatbot language might reduce moral relativity, constrain language interactions, or homogenize language. Students essentially had to think about the language processing constraints in chatbots and how that might affect their language interactions.
Students built chatbots to do everything from helping a student choose colleges for graduate work, college courses, movies, and restaurants, to guiding workouts or choosing a football game to watch. In their subsequent metacognitive reflection assignment, students had plenty to say:
- Chatbots are peculiar devices.
- Chatbots do not process language as humans would.
- Chatbots, because of their limited cognitive capabilities, cannot respond to novel stimuli in conversations and therefore cannot problem-solve or be socially engaging.
- Chatbots have higher potential of providing logical, true and precise answers than humans.
- The nature of chatbot language has positive characteristics that reinvent the notion of interaction, and negative characteristics that create many confusions and misinterpretations about the use of a language.
Using chatbots as a foil prompted students to consider the nature of their own language processes. As a few examples:
For example, human communication is not a mere string of words put together to make meaning, rather it employs many other resources which feed communication such as the extralinguistic, paralinguistic and metalinguistic cues in order to achieve successful communication. I think that chatbots cannot perform such complex task as efficiently as most people do.
Although chatbots may serve humans as they interact with them, I think they do so with a structured sort of language which is intended to perform very specific tasks. As human language is inherently relative and creative, I think chatbots need much improvement to sound like humans if we need them to interact more “naturally.” In terms of human language, a unique characteristic is the ability to process linguistic and non-linguistic inputs. As humans we can process such inputs with the help of our background knowledge, working memory and other brain functions. Our judgements are further constrained, shaped or developed by moral relativity, i.e. the philosophical standpoints given or attributed by the cultures and societies we belong.
The students’ reflections on chatbot language processing fit Flavell’s (1999) suggestion that metacognition includes beliefs about others as cognitive agents, that is, as intelligent communicative actors. Often, learning about metacognitive strategies may begin by observing others and implicitly mimicking their behaviors. For instance, as children we may notice someone writing down a phone number or looking up a phone number and we recognize and adopt these specific processes to manage information. Knowledge of the strategies becomes more explicit the first time we fail to apply the strategy and cannot remember a phone number. We observe classmates reviewing notes repetitively and self-testing and adopt these methods of regulating and monitoring study behaviors. We rarely, if ever, create objects like chatbots, as in the present case, and use the objects to reflect on others’ and our own metacognitive processes, as a learning process. However, as AI technology and products become more prevalent, there arise many natural opportunities to think about and compare machines’ processes to our own. Of course, to qualify as metacognitive thinking, reflections on man vs machine processing will have to go beyond superficial comments like “My Alexa is not too smart.” To be metacognitive, thinking has to be about the processes themselves, in the machine and in the person.
The theme of this post is to highlight how metacognition is not only about thinking about one’s own thinking, but also thinking about thinking in the entities – humans or machines – with whom we communicate. Building a chatbot gives students direct contact with the processes in the machine and a bridge to reflecting on their own processes by comparison. It forces students to reflect on strengths and limitations of both kinds of language. There are other instances where this type of metacognitive knowledge comes into play naturally. Take child-directed speech (a.k.a. motherese, baby talk), for instance. Caretakers adjust their intonation, vocabulary, and rhythm when speaking to infant siblings. They have a sense that an infant is processing language differently so they adjust their own processing to accommodate. Similarly, in the classroom or at a conference, we become aware (sometimes depressingly) that our message is not connecting and may try to make adjustments in speed, terminology, examples, etc. The difference between those situations and the present one is that there may not be a moment of deliberate metacognitive reflection – how is the other person processing information compared to how I am processing the information. Flavell reminds us that this, too, is metacognitive. Here I am suggesting that we can make those moments more deliberate, indeed, we can turn them into class assignments!
References
Draeger, J. (2015). Two forms of ‘thinking about thinking’: metacognition and critical thinking. Retrieved from https://www.improvewithmetacognition.com/two-forms-of-thinking-about-thinking-metacognition-and-critical-thinking/.
Flavell, J. H. (1979). Metacognition and cognitive monitoring: A new area of cognitive–developmental inquiry. American Psychologist, 34(10), 906-911. doi.org/10.1037/0003-066X.34.10.906